Artificial Intelligence Research: Key Innovation Areas and Future Opportunities
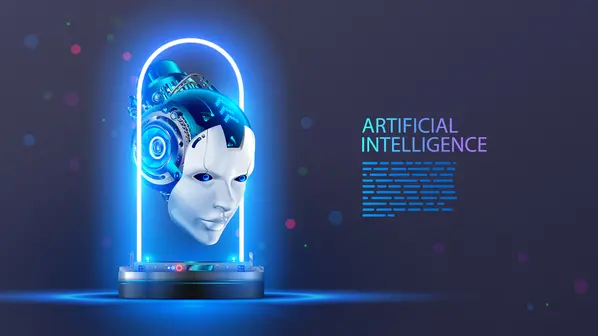
Introduction
Before exploring the future of artificial intelligence (AI), it is essential to understand its definition and current state. AI refers to the capability of machines or computer-operated systems to perform tasks typically associated with human intelligence. The integration of AI with advanced machine learning (ML) methods originating from computer science is significantly influencing various domains, including science and technology, industry, and our everyday lives. ML techniques have been designed to analyze extensive data sets, enabling the extraction of valuable insights, categorization, prediction, and informed decision-making in innovative ways. This progress is paving the way for new applications and supporting the rapid advancement of emerging AI technologies.
Key Opportunities in AI Research
AI research has witnessed a significant revolution during recent times.
AI and Quantum Computing fusion: AI and quantum computing are becoming increasingly popular in business, and they have the tremendous potential to impact ML advancements, a subfield of AI. With the power of quantum computing and mechanics, diverse tasks can be implemented simultaneously. Additionally, AI and quantum computing can handle complicated algorithms faster than traditional computing.
Explainable AI (XAI): XAI is a set of processes and methods that allows users to understand how AI models are making decisions using ML algorithms. With XAI, human users are able to trust the outputs and results generated by ML algorithms. As the demand for understanding AI-based decision-making processes grows, explainable AI facilitates responsible deployment in business contexts, encouraging accountability and fairness within AI innovations, especially when they are put into production.
Reinforcement Learning (RL): RL is an ML training method that assists in decision-making based on learning optimal behavior. This technology engages with the physical environment and studies system responses. In short, RL trains AI agents to observe and interpret their environment. This technology proves effective in diverse environments as long as a clear reward mechanism is in place. Its applications are vast, including training virtual assistants for communication and guiding autonomous robots in navigation tasks.
Advanced Language Modeling Capability: Language modeling capability refers to the ability of a system to observe and comprehend previous language patterns by anticipating the probability of the order of words in a sentence. This allows language translation, spelling correction, speech recognition, text generation, and query resolution based on the context of the input language. This model is the backbone of natural language processing research, or NLP, which helps human users communicate with machines.
Multi-Modal Understanding: Multi-modal AI involves comprehending images and videos for purposes such as detecting deep fakes, identifying visual misinformation, analyzing manipulated scientific texts, and conducting multimedia analyses. It covers face recognition and biometric anti-spoofing measures, as well as enhancing the reliability of AI systems. In addition, there is a focus on understanding AI-powered automation for the extraction of information from countless online tables, with emphasis on automatic layout detection, semantic modeling, retrieval, summarization, entity linking, and fact-checking.
Neural Network and Deep Learning: Deep learning breakthrough is a specialized area within ML. It utilizes deep neural networks, which are composed of multiple layers, to replicate the intricate decision-making capabilities of the human brain. The main difference between deep learning and traditional ML lies in the design of their neural network architectures. Conventional ML models typically feature basic neural networks with one or two layers. In contrast, deep learning models operate with three or more layers, often numbering in the hundreds or thousands, which allows them to perform more advanced training processes.
AI Fairness: AI fairness involves recognizing and addressing bias, enhancing resilience to adversarial attacks, understanding cultural implications, and tackling issues related to polarization and misinformation. Techniques such as forecasting and crowdsourcing are part of this effort.
Challenges and Limitations in AI Research
Some of the challenges faced in AI research include:
- AI Bias
AI systems learn from the data they are fed, and if the data are biased, the AI will adopt those biases. This can result in unfair treatment and discrimination in critical domains such as law enforcement, hiring practices, loan approvals, and more. Therefore, understanding how to implement AI responsibly in these contexts is crucial to minimizing bias. Mitigating AI bias requires a thoughtful approach to data selection, preprocessing methods, and the design of algorithms to enhance fairness.
- AI Integration
Integrating AI into existing processes and systems can be quite complex. This process involves identifying suitable applications for AI, customizing models to fit specific scenarios, and ensuring a smooth integration with current systems. Successful integration necessitates collaboration between AI specialists and industry experts, so that they can thoroughly understand the technologies involved, tailor solutions to meet needs, and achieve organizational goals. Key challenges in this area include data interoperability and the training of personnel, making employee upskilling essential for successful AI adoption.
- Computing Power
AI algorithms demand high-performance computing devices such as GPUs and TPUs. This often results in higher costs and greater energy consumption, presenting significant challenges for smaller organizations. Additionally, emerging technologies like neuromorphic and quantum computing may provide innovative solutions in the early stages of development. Distributed computing and cloud services can be leveraged to address computational limitations. It is crucial to manage computational requirements in a way that balances efficiency and sustainability.
Future Opportunities in AI Innovation
AI is drastically altering the research landscape across disciplines, thus giving rise to multiple opportunities.
- Making AI More Accessible
AI will become a standard tool in personal and professional settings. No-code and low-code platforms will simplify AI adoption, enabling even amateurs to build AI models with drag-and-drop tools. Cloud-based AI services will offer prebuilt models for customization, while open-source collaboration and ethical AI research frameworks will help maintain transparency and security.
- AI Hallucination Insurance
As AI plays a larger role in decision-making, businesses may adopt AI hallucination insurance. This would protect industries like finance, healthcare, and law from risks associated with inaccurate AI-generated insights, similar to data breach policies today.
- AI Regulation and Ethics
Governments will establish stricter guidelines for responsible AI use. Regulations will categorize AI models by risk and impose safeguards on high-risk applications. Ethical concerns will drive bans on harmful AI applications, focusing on transparency, bias reduction, and security.
- Agentic AI
AI systems capable of anticipating needs and making independent decisions will become more prevalent. Agentic AI, which operates using specialized agents, will streamline operations, automate tasks, and enhance customer experiences.
- Emerging AI Technologies
The coming decade may bring breakthroughs in computing architecture. Traditional hardware is reaching its limits, prompting researchers to explore new approaches beyond Moore’s Law. Post-Moore computing will focus on next-generation AI hardware capable of handling increasingly complex tasks, shaping the future of AI integration into daily life and business operations.
Conclusion
AI in scientific discovery is advancing rapidly, opening new possibilities across industries. From quantum computing to explainable AI, innovation is driving smarter, more adaptable systems. As AI becomes more accessible, ethical considerations and regulations will shape its future. The next breakthroughs will define how AI integrates into everyday life, making technology more intuitive and impactful.