The Intersection of Cybersecurity and AI: Trends from Open Access Computer Science Journals
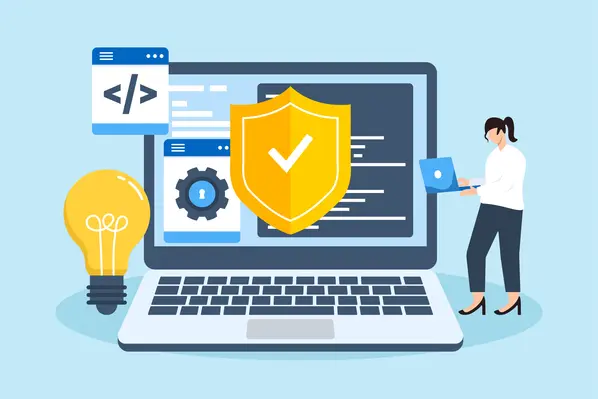
Introduction
The convergence of artificial intelligence (AI) and cybersecurity represents a pivotal technological advancement in the modern digital landscape. Market analysis indicates substantial growth projections, with the global AI cybersecurity market expanding from $22.4 billion in 2023 to an anticipated $60.6 billion by 2028, demonstrating a compound annual growth rate of 21.9%.
AI has transitioned from an auxiliary tool to a fundamental component of contemporary cybersecurity infrastructure. Organizations are implementing sophisticated, adaptive systems that supersede traditional rule-based security protocols, enabling real-time threat identification and response capabilities.
The enhanced threat detection capabilities demonstrate significant technological progress. AI-powered systems present superior efficiency compared to human analysts, processing millions of events concurrently and identifying potential security breaches with unprecedented speed and accuracy. These systems excel in pattern recognition, detecting subtle anomalies that may elude human detection. Contemporary AI-based security platforms incorporate advanced predictive capabilities, adapting defensive measures based on evolving threat patterns. This technological advancement has facilitated a transformation in organizational security strategies, enabling a transition from reactive to proactive defense methodologies.
The implementation of AI in cybersecurity presents both opportunities and challenges. While organizations strengthen their defensive capabilities through AI integration, malicious entities simultaneously incorporate similar technologies into their attack vectors. This dynamic necessitates continuous technological advancement in both defensive and offensive capabilities.
The market statistics, while significant, represent only a quantitative measure of this technological evolution. The broader implication lies in AI's fundamental transformation of cybersecurity frameworks, establishing more robust and adaptive defense systems in response to escalating threat complexity.
AI-Driven Threat Detection and Response
AI has fundamentally altered the capabilities of cybersecurity systems through enhanced detection and automated response mechanisms. Research indicates that there was 72% reduction in false positives and an 88.5% improvement in threat detection accuracy when utilizing AI-driven systems.
Machine learning algorithms, particularly supervised learning models, analyze network traffic patterns to establish behavioral baselines. Machine learning in cybersecurity helps enhance threat detection and response. These systems process multiple data streams simultaneously, including packet metadata, user authentication logs, and system calls. When deviations from established patterns occur, the algorithms flag potential security breaches for investigation.
Deep learning architectures have proven particularly effective in threat prediction. Convolutional Neural Networks (CNNs) process raw network traffic data to identify attack signatures, while Long Short-Term Memory (LSTM) networks analyze sequential data to predict attack vectors.
Several documented cases demonstrate AI's effectiveness in attack mitigation:
-
Financial Sector: A financial institution implemented an AI-based intrusion detection system that identified and blocked a sophisticated Advanced Persistent Threat (APT) attack, preventing data exfiltration within 45 seconds of the initial compromise.
-
Healthcare Industry: A healthcare provider's AI security system detected unusual encryption patterns, halting ransomware propagation across network segments and limiting the impact to 3% of the systems.
-
Telecommunications Field: A telecommunications company's AI-powered threat detection platform identified a zero-day vulnerability exploitation attempt through behavioral analysis, thereby protecting critical infrastructure.
Challenges in Integrating AI into Cybersecurity
The integration of AI into cybersecurity systems involves several significant technical and operational challenges. Security systems face sophisticated adversarial attacks that specifically target AI components through the following:
-
Input manipulation techniques modify malicious code patterns, making them unrecognizable to machine learning models while maintaining their harmful functionality.
-
Advanced data poisoning methods compromise the training process, causing AI systems to learn incorrect patterns and make faulty security decisions.
The autonomous decision-making capabilities of AI systems introduce critical ethical considerations in cybersecurity implementations. These AI cybersecurity challenges manifest in:
-
Accountability gaps when AI systems make autonomous decisions about blocking or allowing network traffic, particularly in cases involving sensitive operations.
-
Complex trade-offs between security and accessibility, where strict AI-driven policies might inadvertently restrict legitimate user activities.
Data privacy represents a fundamental challenge, particularly as organizations implement AI security solutions that require the following:
-
Extensive access to sensitive network traffic data and user behavior patterns for effective threat detection.
-
Storage and processing of proprietary information that must remain protected while being used for AI model training.
The opacity of AI decision-making processes presents substantial operational challenges:
-
Security teams often cannot fully interpret how AI systems identify specific activities as threats, complicating AI-enhanced incident response procedures.
-
The lack of clear audit trails in AI decisions creates difficulties in meeting regulatory requirements and establishing accountability measures.
These challenges underscore the need for developing comprehensive frameworks that address both technical capabilities and governance requirements in AI-driven security implementations.
Current Trends from Open Access Computer Science Journals
AI systems demonstrate particular effectiveness in processing complex security data and identifying sophisticated attack patterns, leading to their increased adoption in organizational security frameworks.
Advanced AI Technologies in Security Operations
The security sector has witnessed significant progress through machine learning and deep learning implementations. These systems excel in threat detection through pattern recognition and behavioral analysis. AI-powered intrusion detection systems now identify network anomalies with heightened precision, while natural language processing enhances protection against social engineering attempts and sophisticated phishing attacks.
Implementation Analysis Through Case Studies
Several implementations demonstrate AI's practical security benefits. In the financial sector, an AI-based detection system identified and neutralized an Advanced Persistent Threat attack in under one minute. The healthcare industry provides another notable example, where AI systems limit ransomware impact to a minimal portion of network infrastructure through early detection. A telecommunications provider successfully prevented zero-day exploitation through AI-driven behavioral analysis.
Quantitative Assessment of AI Security Performance
Performance metrics indicate substantial improvements in security operations through AI integration. Detection systems enhanced with AI capabilities have demonstrated marked improvements in identifying complex attacks compared to conventional methods. The technology shows particular strength in accuracy, with significant reductions in false positive rates while maintaining exceptional threat detection precision. These advancements represent a notable step forward in automated security monitoring capabilities.
Security Implications
Recent findings indicate that AI integration significantly enhances cybersecurity capabilities. The technology demonstrates particular strength in:
-
Rapid identification of complex attack patterns through advanced pattern recognition.
-
Substantial reduction in false positive alerts, improving operational efficiency.
-
Enhanced capability in detecting previously unknown threat vectors.
-
Automated response mechanisms that significantly reduce incident response times.
The consistent advancement in AI security technologies suggests continued improvements in organizational security capabilities, particularly in addressing sophisticated cyber threats.
Future Outlook: AI's Role in Cybersecurity
The future trajectory of AI in cybersecurity indicates substantial technological advancements that will redefine defensive capabilities. Analysis suggests the development of autonomous security systems capable of identifying and neutralizing threats without human intervention. These systems will likely incorporate predictive analytics to address vulnerabilities before exploitation occurs.
The regulatory landscape surrounding AI in cybersecurity continues to mature. Industry experts anticipate comprehensive frameworks that will establish standards for AI security implementations across sectors. These regulations will likely address algorithmic transparency requirements and define clear accountability measures for AI-driven security operations.
Cyber threats display increasing sophistication, combining multiple attack vectors and utilizing AI-powered tools. This evolution necessitates equivalent advancement in defensive capabilities, particularly in:
-
Protection mechanisms for critical infrastructure systems.
-
Advanced detection methods for sophisticated social engineering attempts.
-
Robust defense against coordinated multi-vector attacks.
-
Enhanced capabilities for countering AI-powered offensive tools.
Research priorities focus on developing resilient AI models that maintain effectiveness against emerging threats. Key areas include:
-
AI systems resistant to adversarial manipulation.
-
Enhanced real-time threat detection mechanisms.
-
Advanced authentication systems utilizing AI capabilities.
-
Improved interpretability of AI security decisions.
The progression of cybersecurity threats requires continuous adaptation of defensive strategies. Organizations must maintain current security measures while preparing for emerging challenges through sustained investment in AI security research and implementation.
Conclusion
The integration of AI in cybersecurity represents a significant advancement in organizational defense capabilities. Analysis of current implementations demonstrates substantial improvements in security operations, particularly in threat detection and response mechanisms.
Key findings:
-
Detection systems show a significant improvement in identifying sophisticated attacks.
-
Automated response systems reduce incident handling from hours to minutes.
-
AI implementation challenges center on data privacy and ethical considerations.
-
Regulatory frameworks require careful attention to compliance and transparency.
The security landscape continues to evolve, requiring sustained investment in AI security research and the development of advanced threat detection capabilities. As security challenges grow in complexity, the continued development of AI capabilities remains essential for maintaining effective defense mechanisms. Organizations must prioritize enhanced focus on model resilience and interpretability while adapting to emerging threats.